As your business grows, so does your data, but not always in a good way. Duplicate entries, missing values, and inconsistencies can turn data into a liability rather than an asset. Poor data quality leads to faulty insights, compliance headaches, and wasted time manually fixing errors.
Let's face it, the idea of scaling manual data checks can be daunting and unrealistic. But here’s the good news - automation is here to lighten that load.
In this blog, we’ll break down the must-have features of an automated data quality solution and show how Revefi’s AI-powered tool can help you keep your data clean, accurate, and reliable.
Importance of Data Quality for Business Success
Data quality is fundamental to business success. High-quality data leads to accurate insights, enabling strategic decisions that drive growth. Whereas poor data quality can derail business strategies by leading to the following:
- Faulty Decision Making: Inaccurate data results in misleading analytics and flawed business decisions.
- Increased Compliance Risks: Businesses must adhere to regulatory standards. Poor data quality can lead to compliance issues and hefty fines.
- Wasted Resources: Time and money spent on rectifying errors and managing inefficient processes could be better allocated elsewhere.
So, when you invest in a robust data quality management system, it's not just a technical decision–-it’s a business necessity that empowers you to make confident, data-driven decisions.
Manual vs. Automated Data Quality Management
Traditionally, data quality was maintained through manual checks. Data stewards or IT teams would routinely audit datasets, fix errors, and ensure compliance. However, as the volume and complexity of data have grown, these manual processes have become inefficient and prone to human error.
Manual Data Quality Checks:
- Time-Consuming: Regular audits are labor-intensive and slow.
- Error-Prone: Human oversight can lead to missed anomalies and inconsistencies.
- Not Scalable: As data grows, maintaining manual processes becomes impractical.
Automated Data Quality Solutions:
- Real-Time Monitoring: Automated tools can continuously monitor data streams for errors.
- Scalability: AI-powered platforms can handle massive datasets without a drop in performance.
- Efficiency: Automation reduces manual intervention, freeing up resources for strategic initiatives.
The shift toward automation is critical for organizations looking to maintain high data standards while scaling operations.
Risks Associated with Poor Data Quality
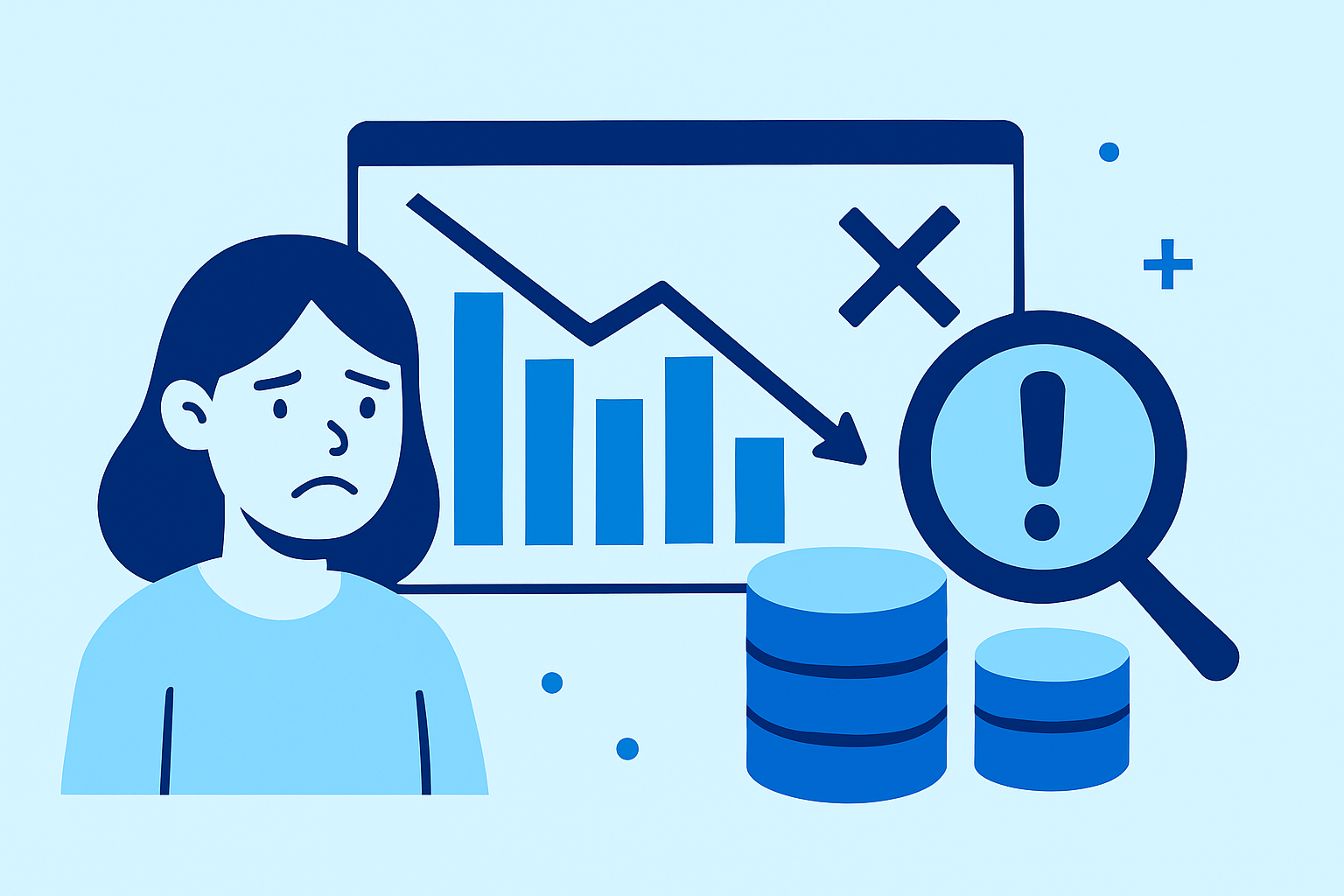
Poor data quality can manifest in several damaging ways:
Inaccurate Analytics & Reporting
Analytics are only as good as the underlying data. Inaccurate data leads to misinformed strategies and poor business outcomes. When analytics are skewed, organizations may invest in the wrong areas, miss emerging trends, or fail to spot opportunities.
Compliance Issues
Regulatory compliance is non-negotiable for modern businesses. With standards such as GDPR and HIPAA in place, inaccurate or incomplete data can result in non-compliance. This not only brings legal consequences but also damages a company’s reputation.
Operational Inefficiencies
Inefficient processes are another consequence of poor data quality. When data is unreliable, teams waste valuable time verifying information, correcting errors, and reconciling discrepancies. This inefficiency can slow down business operations, leading to missed deadlines and lost opportunities.
Key Features to Look for in an Automated Data Quality Solution
When selecting an automated data quality solution, consider the following key features:
Real-time Data Quality Monitoring
Challenge: Many businesses find implementing real-time monitoring across multiple systems difficult, leading to data inconsistencies and delays in issue detection.
Solution: A robust data quality solution continuously tracks data across all sources, ensuring accuracy and consistency. This proactive approach prevents minor errors from escalating into costly problems.
- Continuous Oversight: Automated tools monitor data inputs in real-time.
- Immediate Alerts: The system instantly notifies teams when anomalies are detected.
- Historical Analysis: A record of past issues helps identify recurring patterns and trends.
Enhanced data observability gives you a real-time view of your data's journey across systems, allowing you to detect anomalies and ensure accuracy throughout your operations quickly.
Anomaly Detection
Challenge: Identifying data issues manually is time-consuming and prone to human error, making it difficult to catch inconsistencies before they affect operations.
Solution: AI-powered anomaly detection spots unusual patterns early, ensuring data integrity without requiring manual intervention.
- Pattern Recognition: Machine learning models learn from past data to flag deviations.
- Adaptive Learning: The system improves over time, becoming more accurate in detecting anomalies.
- Actionable Insights: Provides context for errors, making diagnosing and resolving issues easier.
Data Governance
Challenge: Businesses struggle to enforce data policies without proper governance, leading to security risks and compliance issues.
Solution: A strong data governance framework ensures data is handled according to policies and regulations, reducing risks and improving trust.
- Policy Enforcement: Ensures data handling aligns with internal and regulatory standards.
- Traceability: Tracks data movement from source to transformation.
- Audit Trails: Maintains logs of data changes for compliance and troubleshooting.
Cost Optimization
Challenge: Data quality solutions can seem costly upfront, and businesses may struggle to measure their return on investment.
Solution: While there is an initial investment, automation reduces manual work, prevents expensive errors, and ultimately lowers operational costs.
- Resource Efficiency: Automating data tasks frees up employees for strategic work.
- Reduced Downtime: Minimizing errors decreases the need for costly fixes.
- ROI Measurement: The solution should provide insights into cost savings achieved through improved data quality.
Integration with Existing Data Systems
Challenge: Many companies use multiple data systems, making it difficult to implement a data quality solution that seamlessly integrates with all platforms.
Solution: A good data quality solution should work smoothly with existing infrastructure, including data warehouses, CRMs, and BI tools.
- Compatibility: Supports various data sources and formats.
- Ease of Deployment: It can be integrated with minimal disruption.
- Scalability: Adapts to business growth, supporting new data types and systems.
How Leading Companies Use Automated Data Quality Solutions
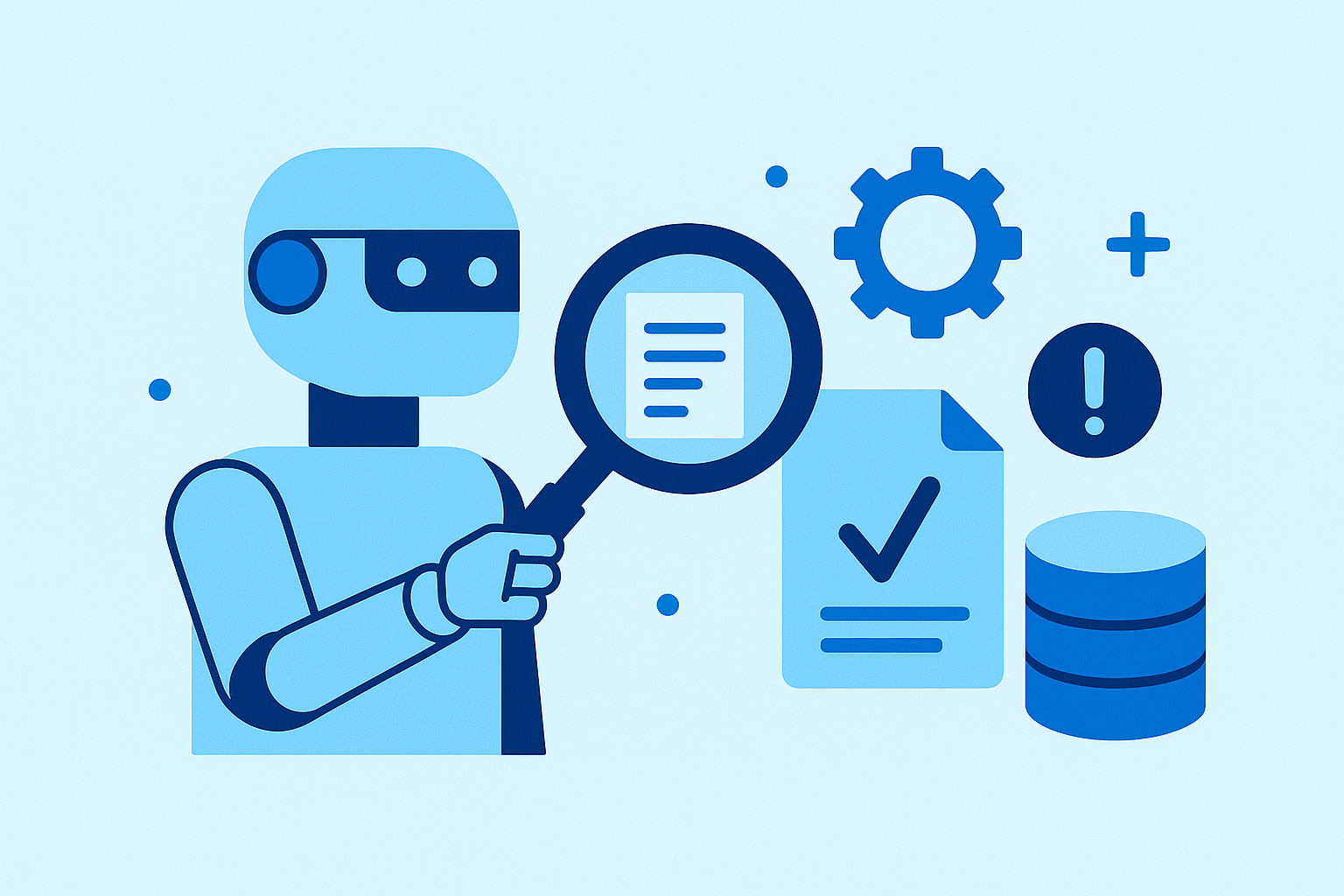
Leading organizations across industries are turning to automated data quality solutions to streamline operations and enhance decision-making. Here are a few examples:
Uplimit
Uplimit, an AI education platform, struggled with maintaining data accuracy, setting up quality monitors manually, and ensuring compliance. By using Revefi, they automated data quality monitoring, eliminating the need for a dedicated resource and gaining better control over compliance and costs.
With a plug-and-play setup, Uplimit achieved 100% automated alerting, saved $10K+ on cloud data warehouse costs, and reduced annual warehouse spending by 50%—all without extra effort.
Tide (UK-based Digital Bank)
Tide simplified GDPR compliance, especially the "Right to be Forgotten." Working with their data and legal teams, they defined personal data and applied these rules across their systems.
They automated data tagging and security, cutting a 50-day manual process down to just a few hours. This made compliance easier and improved data management.
How Revefi Helps You Achieve Reliable Data Quality
Revefi’s AI-powered platform helps growing businesses keep their data accurate and trustworthy. It watches your data around the clock, spots unusual patterns early, and notifies your team so issues can be fixed before they affect decisions.
AI-Driven Monitoring & Anomaly Detection
- Real-Time Checks: The platform continuously checks data to catch problems as they happen.
- Smart Alerts: Its AI learns normal patterns and quickly flags any unusual changes.
Enhanced Accuracy & Scalable Performance
- Adaptive Learning: The system improves its detection over time to keep accuracy high.
- Handles All Sizes: Whether you work with a few records or millions, the platform scales with your needs.
Comprehensive Data Governance
- Clear Policies: It helps enforce data rules and standards across your systems.
- Controlled Access: Role-based controls ensure that only the right people see sensitive data.
- Detailed Logs: Built-in audit trails make it easier to review data changes and meet compliance requirements.
Seamless Integration & Cost Optimization
- Easy Setup: Revefi connects smoothly with your existing data systems without major changes.
- Cost Savings: Cutting down on manual checks saves both time and money on data management.
Maintaining high data quality is essential for sound business decisions. With features like real-time monitoring, smart alerts, and robust governance, Revefi helps you avoid costly errors and keeps your data reliable.
Save Time and Maintain Quality with Revefi
Keeping your data accurate is imperative for any business that wants to succeed today. Moving from manual to automated data quality checks is key to growing without losing accuracy or falling out of compliance.
When you pick an automated solution, look for real-time monitoring, AI-powered anomaly detection, strong data governance, cost-saving features, and easy integration with your current systems.
These features not only reduce risks but also help you make the most of your data. Revefi’s AI platform shows how technology can make data quality management simpler and more reliable. Investing in the right solution today can help prevent expensive mistakes tomorrow and ensure that your business decisions are based on trustworthy information.