Most businesses start with manageable data streams that fit neatly into a handful of spreadsheets. As companies grow, these streams transform into overwhelming rivers of information. The governance approach that worked when your team shared a single office breaks down when you expand across floors, buildings, or continents.
As your company grows, the volume of data can become overwhelming. Data governance, the rules and practices that keep your information accurate, secure, and useful, faces similar growing pains. Manual approaches that serve a startup or small business simply can't keep pace when your data ecosystem expands. It's like trying to manage city traffic with the same stop sign that worked for a quiet neighborhood. But here's the good news-automation can provide relief in this situation, transforming governance into a growth enabler.
This blog explores the common challenges businesses face when scaling data governance, the risks of relying on manual processes, and how automation can transform governance into a growth enabler. You’ll also see real-world examples of companies leveraging automation to improve data quality, compliance, and security.
Challenges of Scaling Data Governance
As organizations expand, their data governance practices face four fundamental obstacles that intensify with growth. These challenges, when not addressed systematically, can lead to what we call a 'governance gap'. This gap widens unless the organization adopts a more scalable and automated approach to data governance.
Inconsistent Data Quality Across Multiple Sources
In growing organizations, different teams often handle data differently. Marketing enters customer information one way, sales another, and support teams develop their own approaches. These differences multiply as businesses add more systems through acquisitions or new technology investments.
The consequences are immediate and frustrating. Monday morning reports show different revenue figures depending on which system generated them. Teams spend hours debating whose numbers are correct rather than making decisions. Executives lose faith in the data, often reverting to gut feelings instead of evidence-based choices.
Compliance Risks
Privacy regulations have evolved from straightforward guidelines to a complex patchwork of requirements. Today's landscape includes GDPR, CCPA, HIPAA, and industry-specific mandates, each demanding specific approaches to handling sensitive information.
When operating in one location, keeping up with local rules remains manageable. As your footprint expands, you're juggling multiple regulatory frameworks with moving compliance targets. Missing these targets isn't just an administrative headache—it risks substantial penalties, operational restrictions, and personal liability for leaders.
Limited Visibility Into Data Usage and Access
The data visibility challenge resembles protecting a home versus securing a sprawling apartment complex. With a house, you know who has keys, when they come and go, and what they access. In a large complex with hundreds of residents, tracking movements becomes exponentially more difficult.
Data environments face similar challenges as they grow. Who downloaded that customer database at midnight? Why is an accounting intern accessing R&D files? Without automated monitoring, these questions often remain unanswered until problems emerge—like discovering a security breach days after it happened.
High Operational Costs
Traditional scaling often follows a linear approach: more data requires more people to manage it. This resembles handling increased mail volume by simply hiring more postal workers—as volume doubles; you need twice the staff; as it triples, three times the workforce.
Many organizations take exactly this approach with data governance, adding specialists for each new data domain or compliance requirement. The economics become unsustainable—data volumes increase exponentially while budgets grow incrementally, creating a widening gap between governance needs and available resources.
Impact of Poor Data Governance on Business Performance
The consequences of inadequate governance extend far beyond technical issues, directly affecting core business functions and results. Organizations experiencing governance shortfalls face three major categories of business impact that undermine their competitive position.
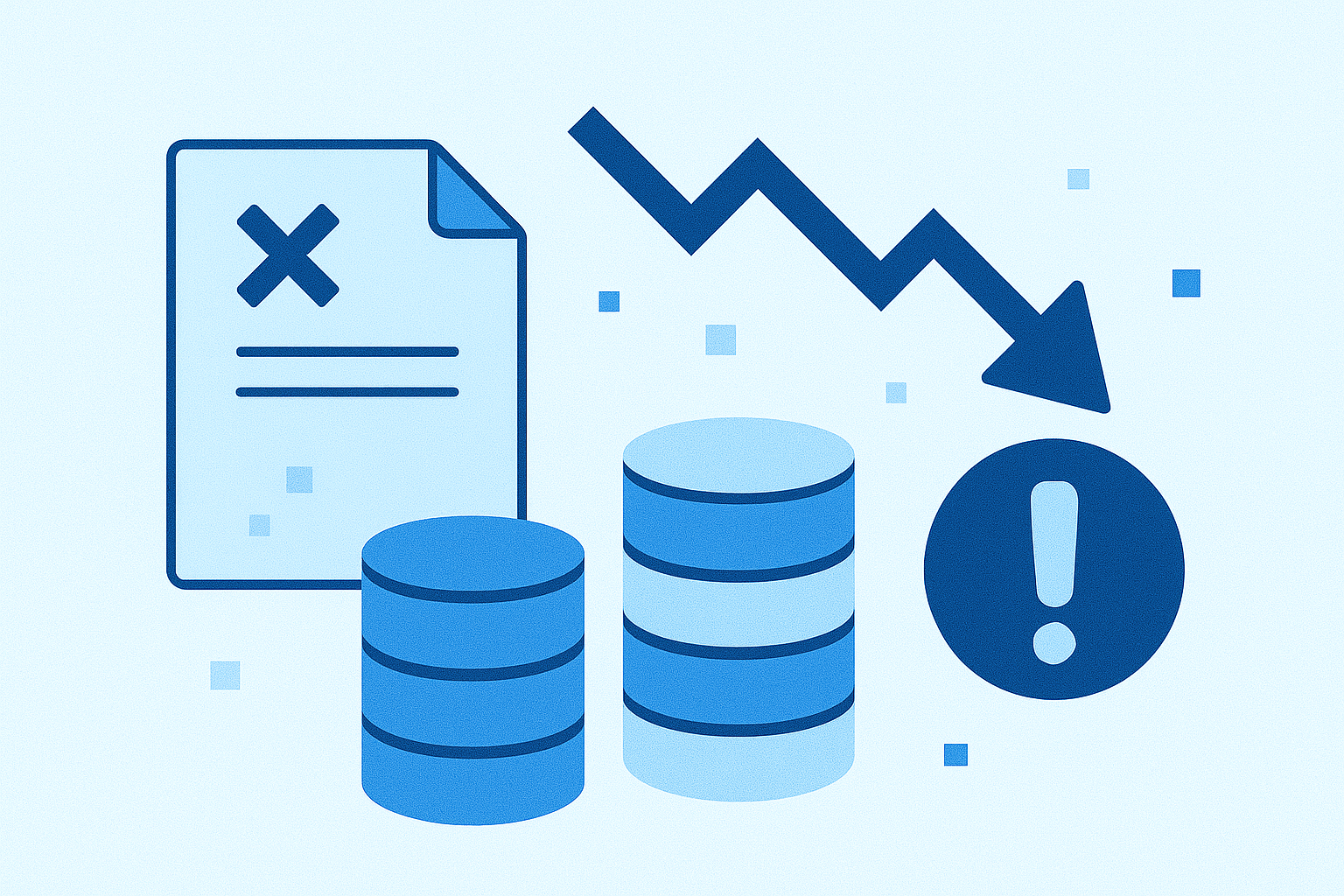
Inefficiencies in Data Access, Security, and Compliance
The frustration of searching for documents you know exist somewhere becomes magnified in organizations with poor data governance. Thousands of employees hunt for critical information across dozens of systems daily.
Research shows knowledge workers spend about a quarter of their time searching for data or reconciling contradictory information—essentially one full day each week on data detective work rather than creating value. Meanwhile, overly complicated security measures lead to workarounds that create bigger problems, like employees emailing sensitive files because the secure sharing system is too cumbersome to use.
Increased Risks of Data Breaches and Non-Compliance Fines
The financial stakes of governance failures have reached unprecedented heights. When sensitive customer information leaks, the average cost now exceeds $4.45 million—a figure that doesn't capture the lost trust and damaged relationships that may never fully recover.
Regulatory penalties add another layer of financial exposure. A significant GDPR violation could cost your company up to 4% of global revenue—potentially millions or even billions of dollars. These aren't theoretical threats but regular headlines affecting companies across industries and size categories.
Slower Decision-Making Due to Unreliable Data
Questionable data quality typically leads to decision paralysis. When faced with contradictory restaurant reviews, most people become hesitant, seek additional information, or default to familiar options rather than trying something new.
Business leaders respond similarly when confronting uncertain data. They delay decisions while requesting additional analysis, choose conservative options to minimize perceived risk, or fall back on intuition rather than leveraging their information assets. In markets where timing often determines success, this hesitation creates tangible competitive disadvantages.
How Automation Transforms Data Governance
Automation is not just a tool, but a fundamental shift in how organizations approach data governance challenges. By applying intelligent technologies to governance processes, companies can achieve levels of consistency, coverage, and cost-effectiveness that are impossible with manual methods. The benefits of automation in data governance are numerous, including improved data quality, enhanced compliance, and increased security. This transformation can significantly improve efficiency and reduce the risk of governance gaps widening.
Data Quality
The evolution in data quality approaches parallels the shift from basic smoke detectors to smart home monitoring systems. Traditional detectors only work when you press the test button, while modern systems continuously monitor conditions and alert you to problems before flames appear.
Automated quality systems monitor information as it enters your ecosystem. Machine learning algorithms recognize patterns indicating potential issues, address missing postal codes or substantial order quantities, and flag them for review before affecting downstream systems. This efficient process ensures that data quality is maintained, allowing your team to focus on more productive tasks.
When European teams enter dates as DD/MM/YYYY while American colleagues use MM/DD/YYYY, these systems automatically standardize formats to prevent confusion. The result shifts organizations from periodic clean-up projects to continuous quality maintenance.
Compliance & Security
The automation of compliance functions resembles how modern vehicles adjust speed when cruise control detects you're getting too close to the car ahead. Both systems continuously monitor conditions against established parameters and take action when needed.
Smart governance systems recognize sensitive information patterns—credit card numbers or medical diagnoses—and automatically apply appropriate protections. When an employee attempts to share protected health information via email, these systems can intervene before the violation occurs, suggesting secure alternatives or triggering approval workflows.
This proactive approach transforms compliance from a retrospective audit exercise to an integrated part of daily operations, preventing violations rather than penalizing them after the fact.
Usage & Governance
The best governance automation works like a perceptive shopping assistant who notices your preferences without explicit instruction. You tend to buy organic produce, so they guide you toward those options; you avoid certain ingredients, so they steer you away from products containing them.
Automated governance tools develop similar insights about data usage patterns. They observe how different teams interact with information, identifying which datasets provide the most value and which security measures create unnecessary friction. These insights allow for contextual governance, applying appropriate controls based on actual usage patterns and risk profiles rather than one-size-fits-all policies.
Data Observability
Modern data observability functions like an air traffic control system tracking every plane's position, altitude, speed, and flight plan simultaneously. Both provide comprehensive visibility across complex environments.
Automated tools trace data from creation through transformation and eventual consumption, documenting lineage and dependencies. When issues arise—perhaps a financial report shows unexpected results—these systems quickly identify which upstream data sources contributed to the problem, dramatically reducing troubleshooting time.
Advanced systems even predict potential problems before they occur, similar to weather forecasting. By analyzing patterns and trends, they identify emerging quality issues or security risks, enabling proactive intervention rather than reactive fixes.
Use Cases: Automation in Action
Theory becomes tangible when we examine how real organizations implement automated governance to address specific challenges. The following examples demonstrate how different industries leverage automation to strengthen governance while scaling their data operations.
1. Financial Services
A wealth management firm struggled with inconsistent client information across their CRM, portfolio management, and reporting systems. After implementing automated data classification and lineage tracking, they reduced regulatory reporting preparation from three weeks to five days while improving accuracy. Their advisors now spend more time with clients and less time reconciling conflicting information.
2. Healthcare Provider Networks
A hospital network faced rising compliance costs and ongoing audit findings related to patient data access. Their automated governance platform now continuously monitors who accesses which records and why, automatically enforcing appropriate permissions while generating HIPAA compliance documentation. The compliance team reduced their overhead by a third while strengthening patient data protection.
3. Retail and E-commerce
A retailer selling through their website, mobile app, and third-party marketplaces struggled with product information consistency. Their automated quality monitoring system now catches discrepancies in pricing, descriptions, and availability in real-time. Customer complaints about incorrect information dropped by 40%, while the team maintaining product data cut their workload significantly.
How Revefi Empowers Scalable Data Governance
While many solutions address specific governance challenges, Revefi takes a comprehensive approach that integrates advanced capabilities into a cohesive platform. Their technology specifically targets the pain points encountered by growing organizations attempting to scale their governance practices.
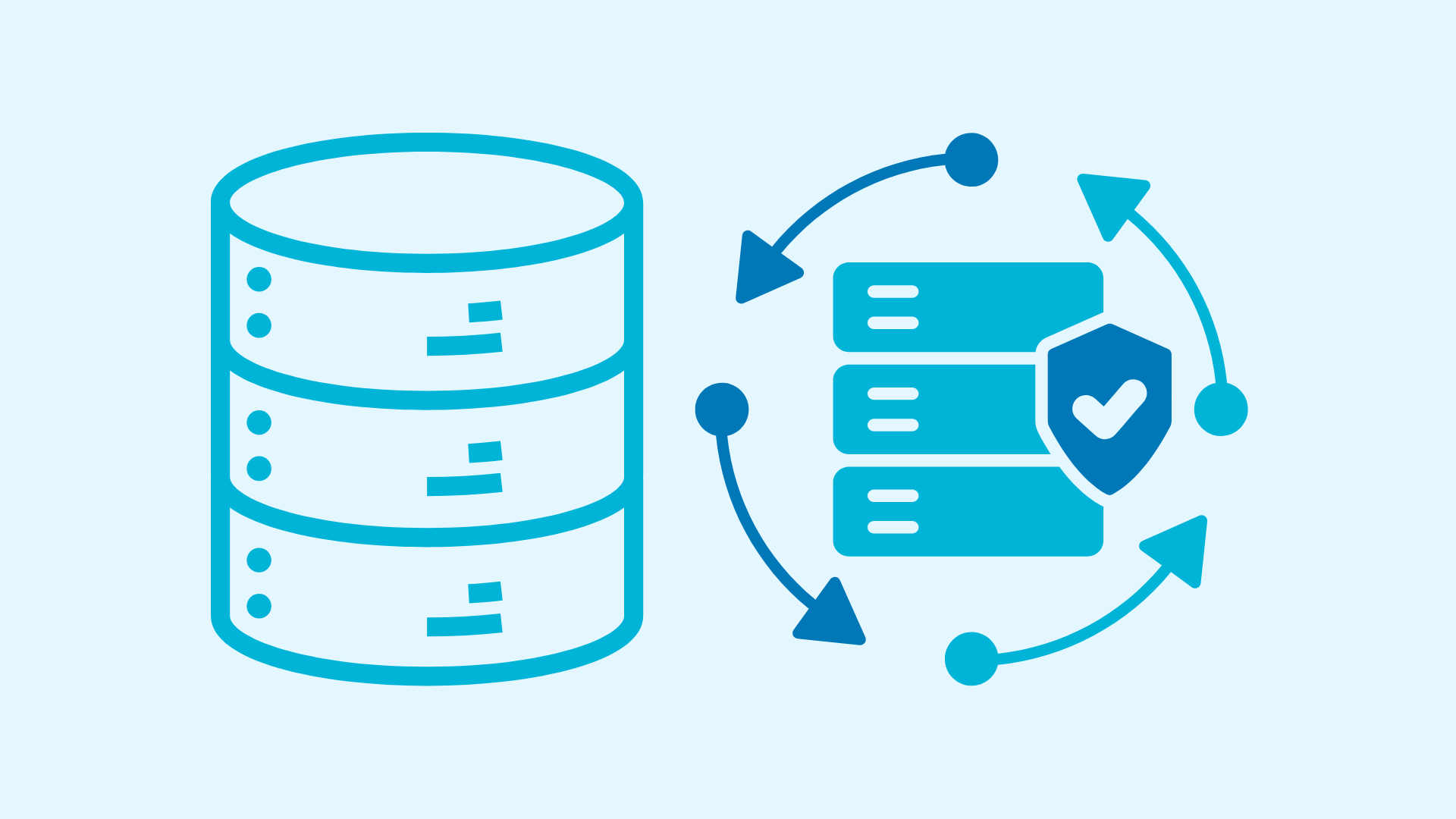
AI-Driven Governance: Revefi's intelligence capabilities work like a seasoned governance professional who learns your business needs over time. The platform observes data patterns, user behaviors, and compliance requirements to create an adaptive framework that evolves with your organization. This approach recognizes that governance isn't one-size-fits-all—what works for financial data might be inappropriate for marketing content.
Automated Compliance: Revefi transforms compliance from a dreaded audit exercise to a continuous background function. The platform keeps pace with regulatory changes across jurisdictions, automatically connecting requirements to your specific data assets and controls. When regulations evolve, the system identifies affected information and processes, removing the periodic scramble to address new requirements.
Related: Data Governance and Management for ThoughtSpot
Real-time Observability: Revefi provides the visibility that governance teams have always wanted but rarely achieved. Interactive dashboards display quality metrics, access patterns, compliance status, and potential issues as they develop. This clarity helps data stewards shift from reactive problem-solving to strategic management of information assets.
Scalable Architecture: Unlike point solutions that create governance silos, Revefi's platform grows with your business. Whether you're adding new data sources, expanding into new markets, or integrating acquisitions, the governance capabilities scale alongside your operations rather than becoming bottlenecks to growth.
Conclusion
The manual data governance approaches that served businesses adequately in the past cannot keep pace with today's explosive data growth. Organizations trying to scale these traditional methods face mounting costs, growing risks, and diminishing returns.
Automation offers a better path forward—not by replacing human judgment but by amplifying it. Smart systems handle repetitive monitoring and enforcement tasks, freeing governance professionals to focus on strategy and complex decisions that require human insight.
Revefi’s approach helps bridge the gap between governance aspirations and practical implementation. By combining artificial intelligence, automated compliance checking, and continuous observability, the platform helps maintain data integrity and security without creating innovation bottlenecks.
Want to see automated data governance in action? Watch a live demo or explore how Revefi's AI-driven solutions can transform your data strategy today.